Accepted submissions
Accepted papers in both the extended abstract and proceedings track of GRaM can be found here!
Accepted blogposts and tutorials can be found here!
Motivation
By recognizing that nearly all data is rooted in our physical world, and thus inherently grounded in geometry and physics, it becomes evident that representation learning should preserve this grounding in order to remain meaningful. For example, preserving group transformation laws and symmetries through equivariant layers is crucial in domains such as computational physics, chemistry, robotics, and medical imaging, and leads to effective and generalizable architectures and improved data efficiency. Similarly, in generative models applied to non-Euclidean data spaces, maintaining the manifold structure is essential in order to obtain meaningful samples. Therefore, this workshop focuses on the principle of grounding in geometry, which we define as follows:
A representation, method, or theory is grounded in geometry if it can be amenable to geometric reasoning, that is, it abides by the mathematics of geometry and physics.
Topics
We solicit submissions that present theoretical research, methodologies, applications, insightful analysis, and even open problems, within the following topics (list not exhaustive):
- Structure-preserving learning
- Preservation of symmetries; E.g., through equivariant operators.
- Dynamical systems on manifolds; Representation learning and generative modeling using ordinary, stochastic, and differential equations (ODEs, SDEs, PDEs) on manifolds.
- Computing with geometric representations; Such as the processing of multi-vectors using geometric algebra, steerable vectors using Clebsch-Gordan products, and hyperbolic features using Fréchet means.
- Structure-inducing learning
- Self-supervised learning; E.g., learning to embed data in geometric latent spaces through (geodesic) distance-based similarity metrics.
- Geometric priors; E.g., soft constraints on model weights.
- Physics-Informed Neural Networks; E.g., inducing the structure of established physical and geometric laws into neural networks through dedicated losses.
- Generative modeling and density estimation
- Geometric latent variable models; I.e., the use of latent variables that live in a manifold.
- New Methods; And adaptations of methods capable of:
- Generating geometric objects; E.g., generating atomic point clouds or shapes.
- Generating fields over manifolds; E.g., generating vector fields or spherical signals.
- Grounding in theory
- Theoretical frameworks; Unifying analyses and formulations that provide a generalizing perspective on deep learning paradigms.
- Open problems; Identifying and addressing unresolved questions and challenges that lie at the intersection of geometry and learning
Call for papers
The workshop solicits submissions to the
- Proceedings track: 8 page paper submissions (excluding appendices and references). Submissions will be peer-reviewed. Accepted papers will be published in PMLR as part of our workshop proceedings.
- Extended abstract track: 4 page paper submissions (excluding appendices and references). Submissions will be peer-reviewed. Accepted papers can be viewed on openreview.
- Blogpost track: blog posts that have tutorial value and act as explainers for (previously) published papers or important topics in the field. In this track, we further encourage blog posts that present opinion pieces, open problems,etc in provided markdown format. Submissions will be peer-reviewed and accepted submissions will be hosted on GRaM wesbite.
- Tutorial track : Tutorials with easy-to-use and understand code for topics of important to GRaM audience submitted as a collab file. Submissions will be peer-reviewed and accepted submissions will be hosted on GRaM website.
- TAG challenge: We team up with TAG and host a ICML Topological Deep Learning Challenge 2024: Beyond the Graph Domain challenge.
Reviewing for GRaM
If you would like to review for our workshop, please signup here.
Contact
If you have any questions, please feel free to email us at organizers AT gram-workshop DOT org
Organizers
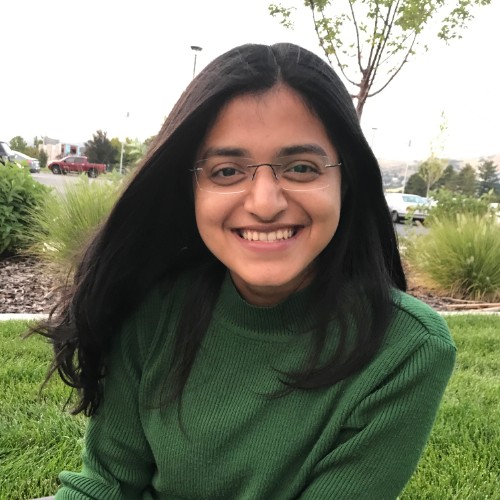
Sharvaree Vadgama is a PhD student at Amsterdam Machine Learning Lab, University of Amsterdam. Her work is focused on learning representations primarily in the intersection of geometric deep learning and generative modeling. She was a co-organizer of Generative Modeling Summer School (GeMSS) 2023 as well as a co-organizer of Women in AI meetups (Amsterdam chapter).
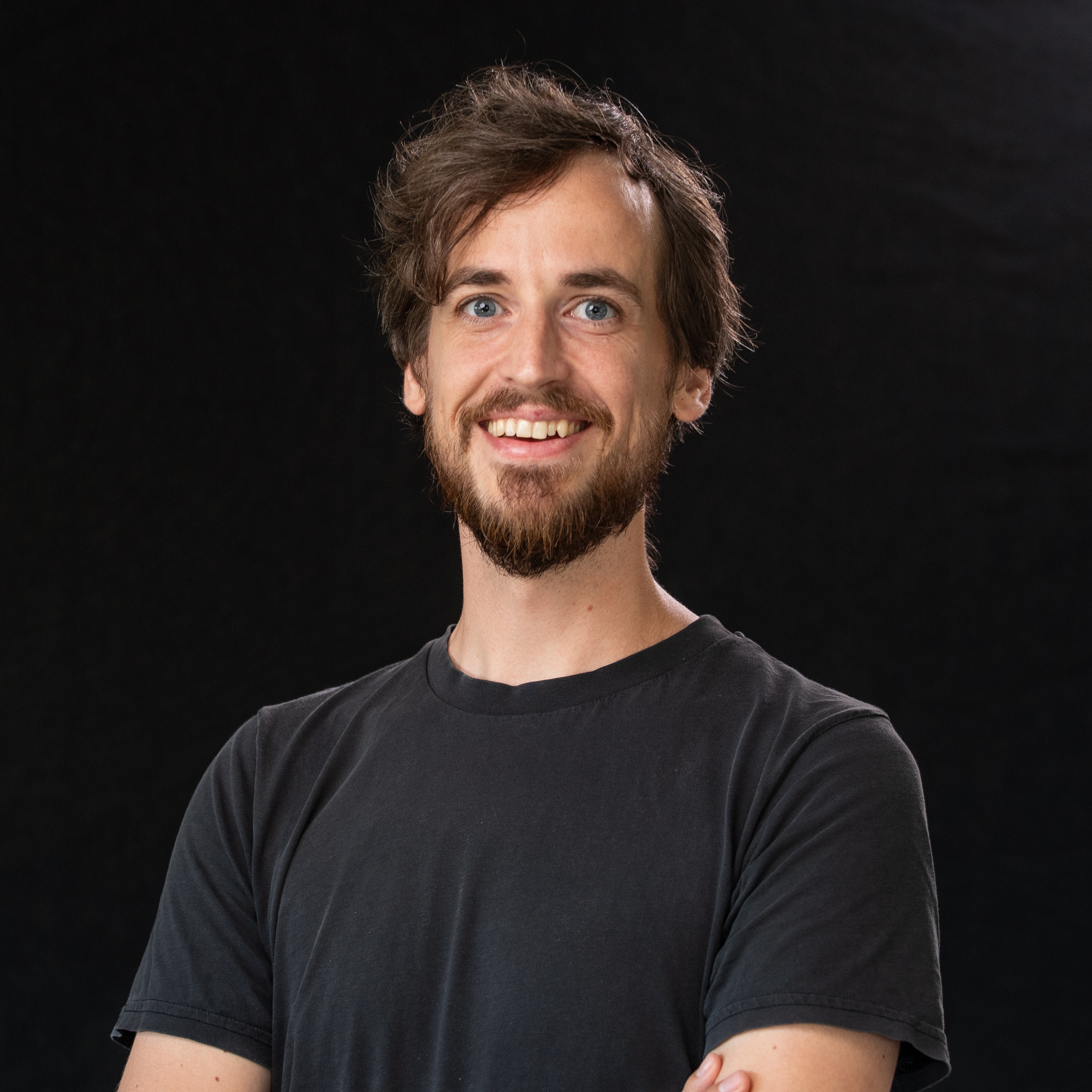
Erik Bekkers is an assistant professor at the Machine Learning Lab of the University of Amsterdam (AMLab, UvA) and an ELLIS Scholar in the program on Geometric Deep Learning. His research is equivariant deep learning and computational and representation efficiency through sparse, adaptive, and geometric learning mechanisms. He has organized several international workshops and conference sessions (e.g., GeoMedIA'22, GSI '21 and '23, two ELLIS workshops on GDL). He chairs the board of examiners for the Master AI at UvA. Erik received a VENI personal research grant (Dutch Research Council) and the Young Scientist Award at MICCAI 2018.
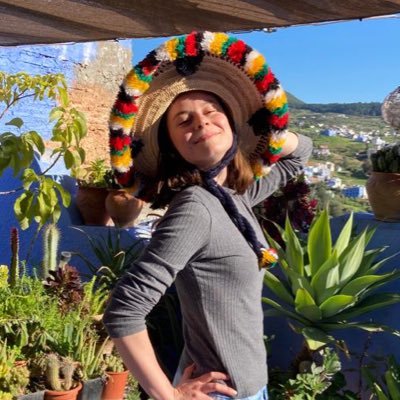
Alison Pouplin is a postdoctoral researcher at the Finnish Center of AI. Her current research interests involve geometric deep learning and generative modeling applied to drug discovery. She defended her PhD on differential geometric approaches to machine learning.
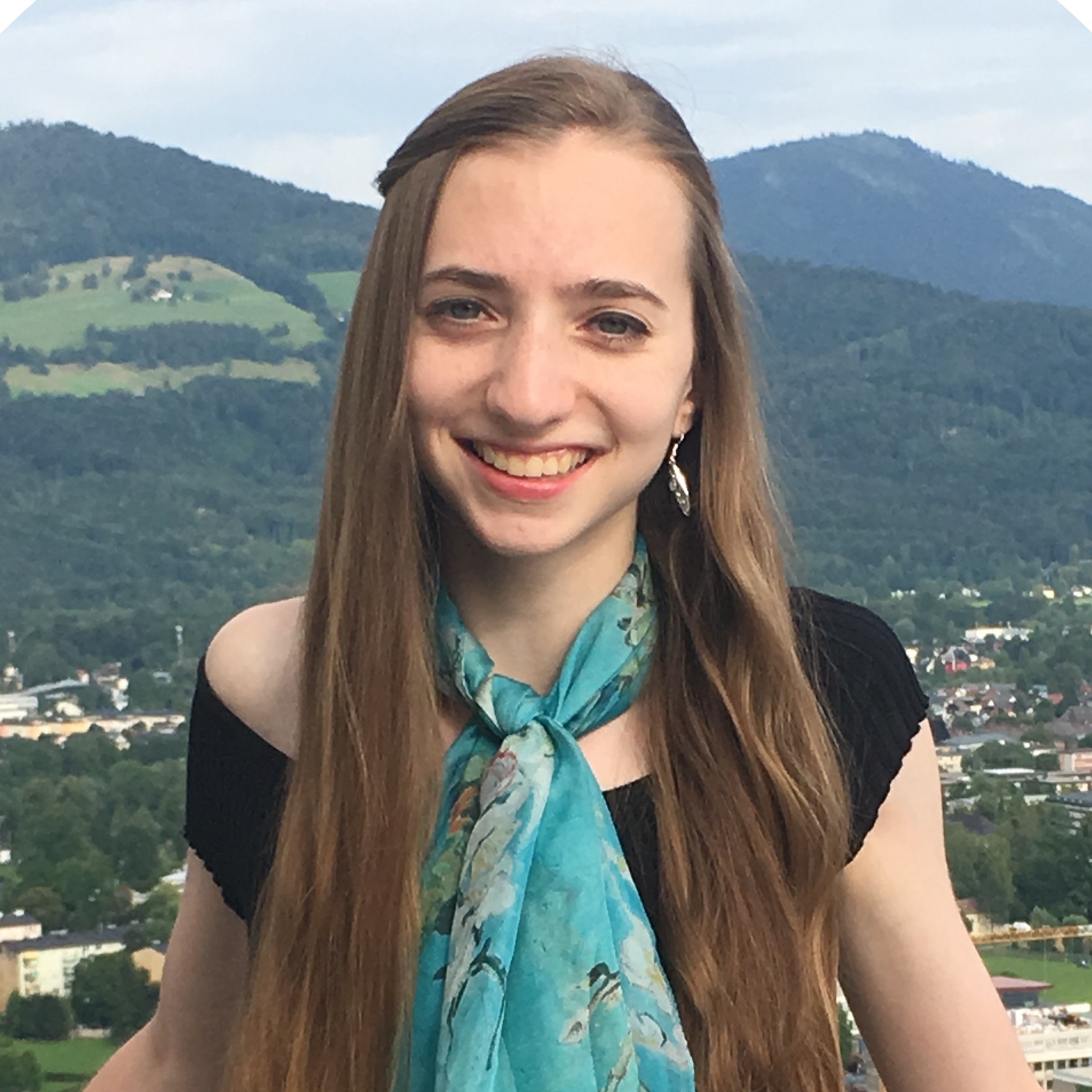
Hannah Lawrence is a PhD student at the Massachusetts Institute of Technology. Her research centers on symmetries in deep learning, aiming to both establish theoretical foundations and efficiently exploit equivariance in (often scientific) applications. She is also a co-founder of the Boston Symmetry Group, which organizes an annual workshop for Boston-area researchers in equivariance. She is supported by the Hertz Foundation and the National Science Foundation.
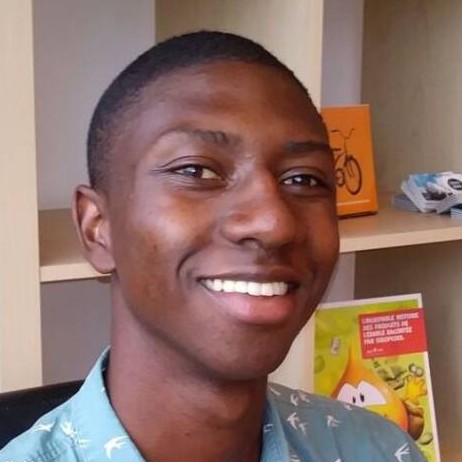
Sékou-Oumar Kaba is a PhD student at McGill University and Mila, the Quebec AI institute. His interests are at the intersection of physics and deep learning, with a focus on symmetry and geometric inductive biases. In parallel, he also works on applications of machine learning to material science. He is a co-organizer of the Mila Quantum and AI meetups. He is a recipient of the DeepMind PhD scholarship.
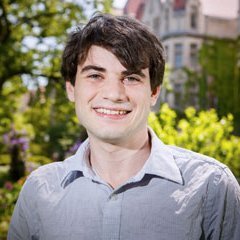
Robin Walters is an assistant professor in the Khoury College of Computer Sciences at Northeastern University, where he leads the Geometric Learning Lab. Robin’s research seeks to develop a fundamental understanding of the role symmetry plays in deep learning and to exploit this to improve the generalization and data efficiency of deep learning methods. This includes designing equivariant neural networks, symmetry discovery methods, and creating a theory of symmetry for model parameters. He has applied these methods to improve models in domains with complex dynamics including climate science, transportation, and robotics.
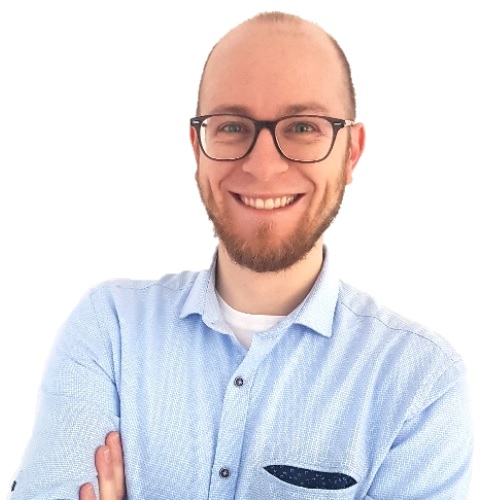
Jakub M. Tomczak is an associate professor and the PI of the Generative AI group at the Eindhoven University of Technology (TU/e). His main research interests include deep generative modeling, deep learning, and Bayesian inference, with applications to image/text processing, Life Sciences, and Molecular Sciences. He serves as an action editor of "Transactions of Machine Learning Research", and an area chair of major AI conferences (e.g., NeurIPS, ICML, AISTATS). He was a co-organizer of the Neurosymbolic and Generative Models (NeSy-GeMs) Workshop at ICLR 2023. He will serve as a program chair of NeurIPS 2024. He is the author of the book entitled "Deep Generative Modeling", the first comprehensive book on Generative AI. He is also the founder of Amsterdam AI Solutions.
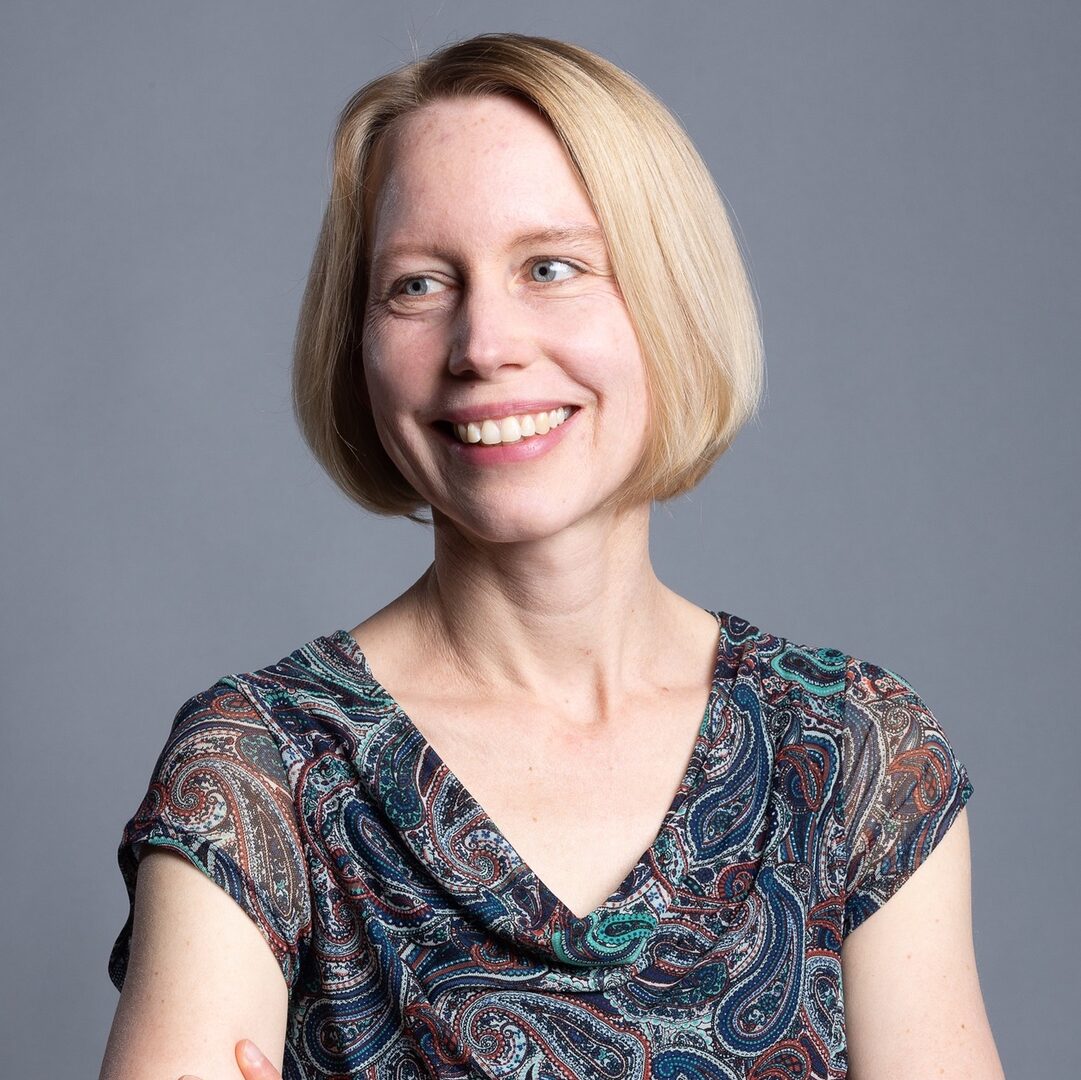
Stefanie Jegelka is an Associate Professor (on leave) at MIT EECS, and a Humboldt Professor at TU Munich. At MIT, she is a member of CSAIL, IDSS, and the Center for Statistics and Machine Learning. Before that, she was a postdoc in the AMPlab and computer vision group at UC Berkeley. Her research is in algorithmic machine learning and spans modeling, optimization algorithms, theory, and applications. In particular, her team has been working on exploiting mathematical structure for discrete and combinatorial machine learning problems, for robustness, and for scaling machine learning algorithms.
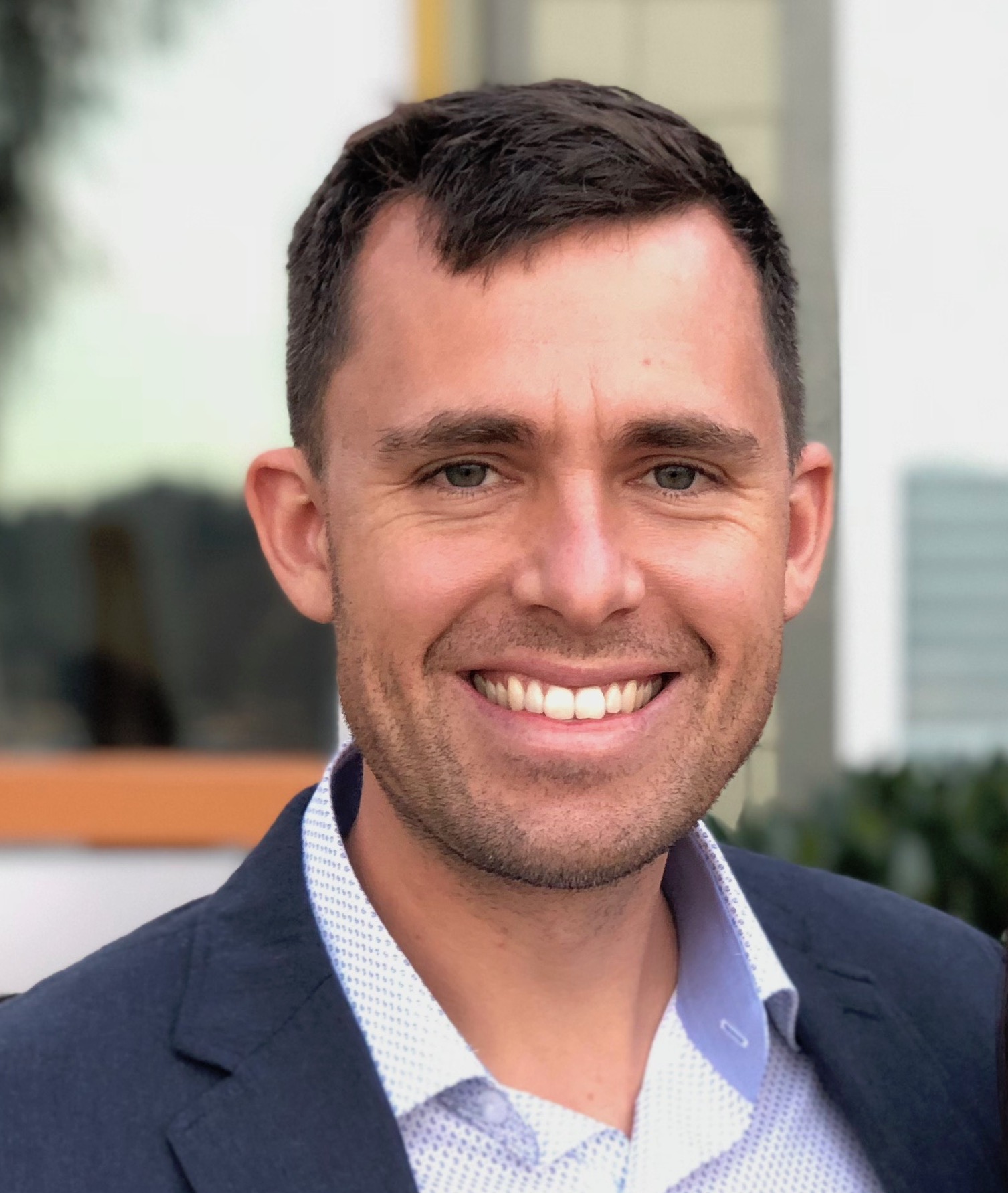
Henry Kvinge is a mathematician and machine learning researcher at Pacific Northwest National Lab and an Affiliate Assistant Professor in the University of Washington Mathematics Department. His research interests include representation learning, improving the robustness of deep learning models, and applications of topology, algebra, and geometry to machine learning and data science. He also works on applying machine learning to materials science. Henry organizes the 'Pacific Northwest Seminar on Topology, Algebra, and Geometry in Data Science' and is one of the founding organizers of the Topology, Algebra, and Geometry in Data Science series. His work has been featured in several articles, including recent papers on hidden activations in Stable Diffusion and the use of AI in advanced manufacturing processes.
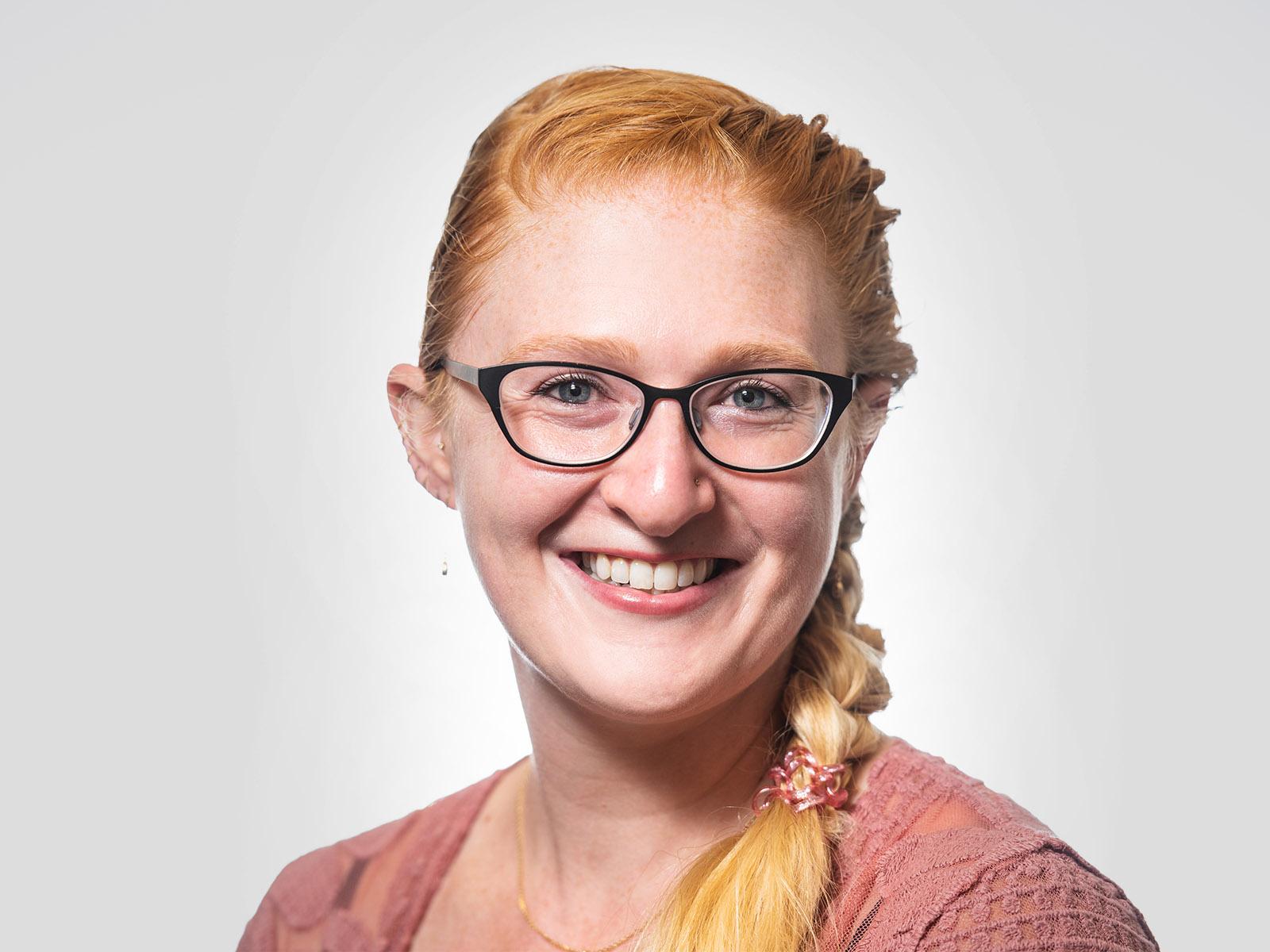
Tegan Emerson received her PhD in Mathematics from Colorado State University. She was a Jerome and Isabella Karle Distinguished Scholar Fellow in optical sciences at the Naval Research Laboratory from 2017-2019. In 2014 she had the honor of being a member of the American delegation at the Heidelberg Laureate Forum. Dr. Emerson is now a Senior Data Scientist and Team Leader in the Data Sciences and Analytics Group at Pacific Northwest Laboratory. In addition to her role at Pacific Northwest National Laboratories, Dr. Emerson also holds Joint Appointments as Affiliate Faculty in the Departments of Mathematics at Colorado State University and the University of Texas, El Paso. Her research interests include geometric and topological data analysis, dimensionality reduction, algorithms for image processing and materials science, deep learning, and optimization.